Prior and (noisy) posterior predictions#
Show the difference between noisy and noiseless predictions by plotting. Now we generate 1\(D\) toy data \((x_i\in\mathbb R, y_i\in\mathbb R)\) and also perform a “real GP fit” by optimizing the \(\ell\) hyperparameter. However we fix \(\sigma_n^2\) to certain values to showcase the different noise cases.
Below \(\ma\Sigma\equiv\cov(\ve f_*)\) is the covariance matrix from [RW06] eq. 2.24 with \(\ma K = K(X,X)\), \(\ma K'=K(X_*, X)\) and \(\ma K''=K(X_*, X_*)\), so
Imports and helpers
Show code cell source
from functools import partial
from box import Box
import numpy as np
from sklearn.gaussian_process import GaussianProcessRegressor
from sklearn.gaussian_process.kernels import RBF, WhiteKernel
from sklearn.preprocessing import StandardScaler
import matplotlib.pyplot as plt
from matplotlib import is_interactive
from common import (
textbook_prior,
textbook_posterior,
textbook_posterior_noise,
cov2std,
sample_y,
)
plt.rcParams["figure.autolayout"] = True
plt.rcParams["font.size"] = 18
def transform_labels(name: str):
lmap = dict(
noise_level=r"$\sigma_n^2$",
length_scale=r"$\ell$",
y_std_pn=r"$\sqrt{\mathrm{diag}(\Sigma)}$",
y_std_p=r"$\sqrt{\mathrm{diag}(\Sigma + \sigma_n^2\,I)}$",
y_mean=r"$\mu$",
)
return lmap.get(name, name)
def gt_func(x):
"""Ground truth"""
return np.sin(x) * np.exp(-0.1 * x) + 10
def transform_1d(scaler: StandardScaler, x):
assert x.ndim == 1
return scaler.transform(x.reshape(-1, 1))[:, 0]
def calc_gp(
*,
pri_post: bool,
noise_level: float,
pred_mode: str,
XI: np.ndarray,
X: np.ndarray = None,
y: np.ndarray = None,
):
if pri_post == "pri":
length_scale = 0.5
gp = GaussianProcessRegressor(
kernel=RBF(length_scale=length_scale)
+ WhiteKernel(noise_level=noise_level),
alpha=0,
normalize_y=False,
)
elif pri_post == "post":
gp = GaussianProcessRegressor(
kernel=RBF(length_scale_bounds=[1e-5, 10])
+ WhiteKernel(noise_level=noise_level, noise_level_bounds="fixed"),
n_restarts_optimizer=5,
alpha=0,
normalize_y=False,
)
gp.fit(X, y)
length_scale = gp.kernel_.k1.length_scale
# gp.kernel_.k2.noise_level == noise_level (fixed)
else:
raise ValueError(f"unknown {pri_post=}")
y_mean, y_cov = gp.predict(XI, return_cov=True)
if pred_mode == "p":
post_ref_func = textbook_posterior_noise
elif pred_mode == "pn":
post_ref_func = textbook_posterior
y_cov -= np.eye(XI.shape[0]) * noise_level
else:
raise ValueError(f"unknown {pred_mode=}")
y_std_label = transform_labels(f"y_std_{pred_mode}")
y_std = cov2std(y_cov)
if pri_post == "pri":
y_mean_ref, y_std_ref, y_cov_ref = textbook_prior(
noise_level=noise_level, length_scale=length_scale
)(XI)
else:
y_mean_ref, y_std_ref, y_cov_ref = post_ref_func(
X, y, noise_level=noise_level, length_scale=length_scale
)(XI)
np.testing.assert_allclose(y_mean, y_mean_ref, rtol=0, atol=1e-9)
np.testing.assert_allclose(y_std, y_std_ref, rtol=0, atol=1e-9)
np.testing.assert_allclose(y_cov, y_cov_ref, rtol=0, atol=1e-9)
samples = sample_y(y_mean, y_cov, 10, random_state=123)
if pri_post == "pri":
if noise_level == 0:
cov_title = r"$\Sigma=K''$"
else:
cov_title = r"$\Sigma=K'' + \sigma_n^2\,I$"
else:
if noise_level == 0:
cov_title = r"$\Sigma=K'' - K'\,K^{-1}\,K'^\top$"
else:
cov_title = r"$\Sigma=K'' - K'\,(K+\sigma_n^2\,I)^{-1}\,K'^\top$"
cov_title += "\n" + rf"$\sigma$={y_std_label}"
return Box(
y_mean=y_mean,
y_cov=y_cov,
y_std=y_std,
samples=samples,
cov_title=cov_title,
length_scale=length_scale,
noise_level=noise_level,
y_std_label=y_std_label,
pri_post=pri_post,
)
def plot_gp(
*, box: Box, ax, xi, std_color="tab:orange", x=None, y=None, set_title=True
):
if set_title:
ax.set_title(
f"{transform_labels('noise_level')}={box.noise_level} "
f"{transform_labels('length_scale')}={box.length_scale:.5f}"
"\n" + box.cov_title
)
samples_kind = "prior" if box.pri_post == "pri" else "posterior"
for ii, yy in enumerate(box.samples.T):
ax.plot(
xi,
yy,
color="tab:gray",
alpha=0.3,
label=(f"{samples_kind} samples" if ii == 0 else "_"),
)
ax.plot(
xi,
box.y_mean,
lw=3,
color="tab:red",
label=transform_labels("y_mean"),
)
if box.pri_post == "post":
ax.plot(x, y, "o", ms=10)
ax.fill_between(
xi,
box.y_mean - 2 * box.y_std,
box.y_mean + 2 * box.y_std,
alpha=0.1,
color=std_color,
label=rf"$\pm$ 2 {box.y_std_label}",
)
Generate 1\(D\) toy data
Show code cell source
seed = 123
rng = np.random.default_rng(seed=seed)
x = np.sort(rng.uniform(0, 5, 5), axis=0)
xspan = x.max() - x.min()
xi = np.linspace(x.min() - 0.3 * xspan, x.max() + 0.3 * xspan, len(x) * 50)
y = gt_func(x)
in_scaler = StandardScaler().fit(x.reshape(-1, 1))
out_scaler = StandardScaler().fit(y.reshape(-1, 1))
x = transform_1d(in_scaler, x)
xi = transform_1d(in_scaler, xi)
y = transform_1d(out_scaler, y)
X = x[:, None]
XI = xi[:, None]
noise_level = 0.3
y_lim = (-3, 3)
Prior, noiseless#
First we plot the prior, without noise (predict_noiseless
).
This is the standard textbook case. We set \(\ell\) to some constant of our liking since its value is not defined in the absence of training data.
Show code cell source
# -----------------------------------------------------------------------
# prior
# -----------------------------------------------------------------------
figsize_single = (10, 9)
d_pri_0 = calc_gp(
pri_post="pri",
noise_level=0,
pred_mode="pn",
XI=XI,
)
fig, ax = plt.subplots(figsize=figsize_single)
plot_gp(box=d_pri_0, ax=ax, xi=xi)
_ = ax.set_ylim(*y_lim)
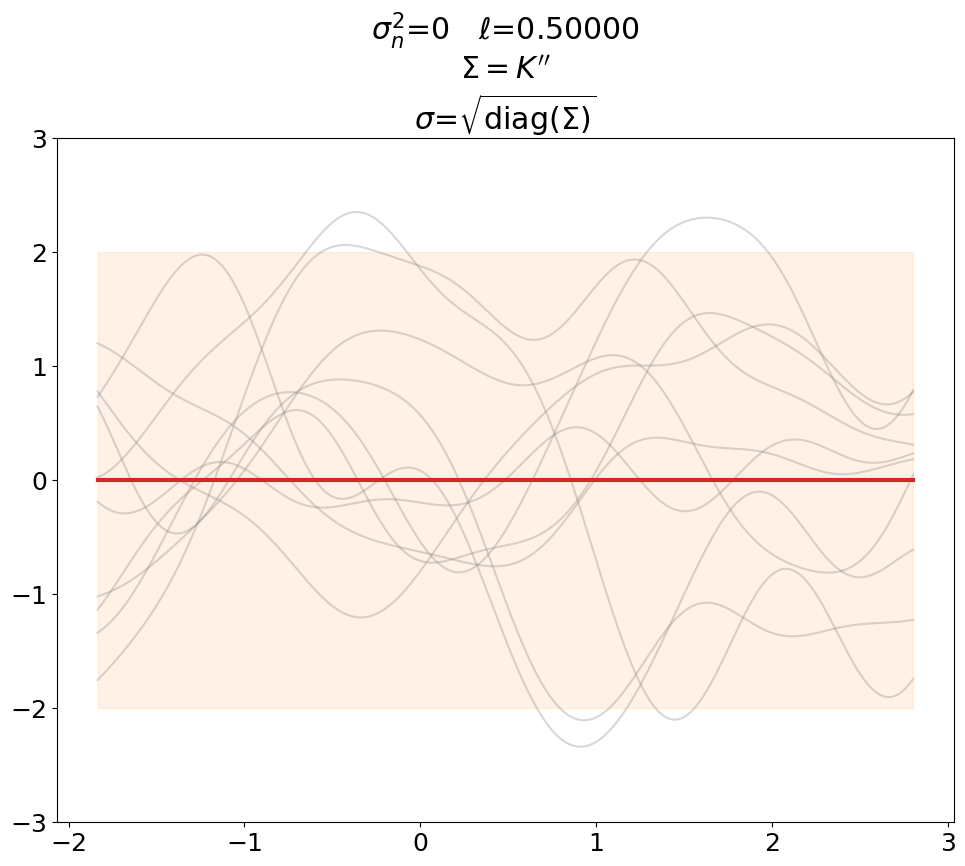
Prior, noisy#
Even though not super useful, we can certainly generate noisy prior samples
in the predict
setting when using \(\ma K'' + \sigma_n^2\,\ma I\) as prior
covariance.
This is also shown in [DLvdW20] in fig. 4.
Show code cell source
d_pri_p = calc_gp(
pri_post="pri",
noise_level=noise_level,
pred_mode="p",
XI=XI,
)
fig, ax = plt.subplots(figsize=figsize_single)
plot_gp(box=d_pri_p, ax=ax, xi=xi)
_ = ax.set_ylim(*y_lim)
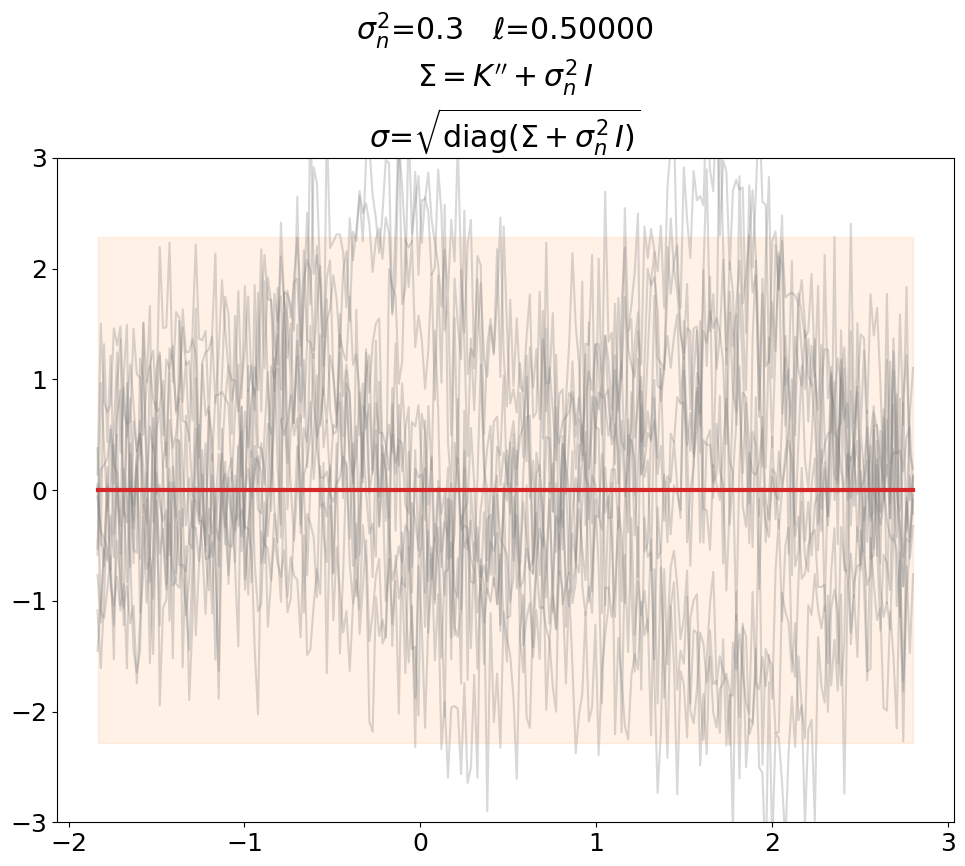
Posterior, noiseless, interpolation#
Now the posterior.
For that, we do an \(\ell\) optimization using 1\(D\) toy data and sklearn
with fixed \(\sigma_n^2\):
interpolation (\(\sigma_n = 0\))
regression (\(\sigma_n > 0\))
Show code cell source
# -----------------------------------------------------------------------
# posterior
# -----------------------------------------------------------------------
calc_gp_post = partial(calc_gp, X=X, y=y, XI=XI)
d_post_0 = calc_gp_post(
pri_post="post",
noise_level=0,
pred_mode="pn",
)
d_post_p = calc_gp_post(
pri_post="post",
noise_level=noise_level,
pred_mode="p",
)
d_post_pn = calc_gp_post(
pri_post="post",
noise_level=noise_level,
pred_mode="pn",
)
y_std_p_color = "tab:cyan"
y_std_pn_color = "tab:orange"
plot_gp_post = partial(plot_gp, x=x, y=y, xi=xi)
Interpolation (\(\sigma_n^2=0\)).
This is a plot you will see in most text books. Notice that \(\ell\) is not 0.5 as above but has been optimized (maximal LML).
Show code cell source
fig, ax = plt.subplots(figsize=figsize_single)
plot_gp_post(box=d_post_0, ax=ax)
ax.legend(loc="upper right")
_ = ax.set_ylim(*y_lim)
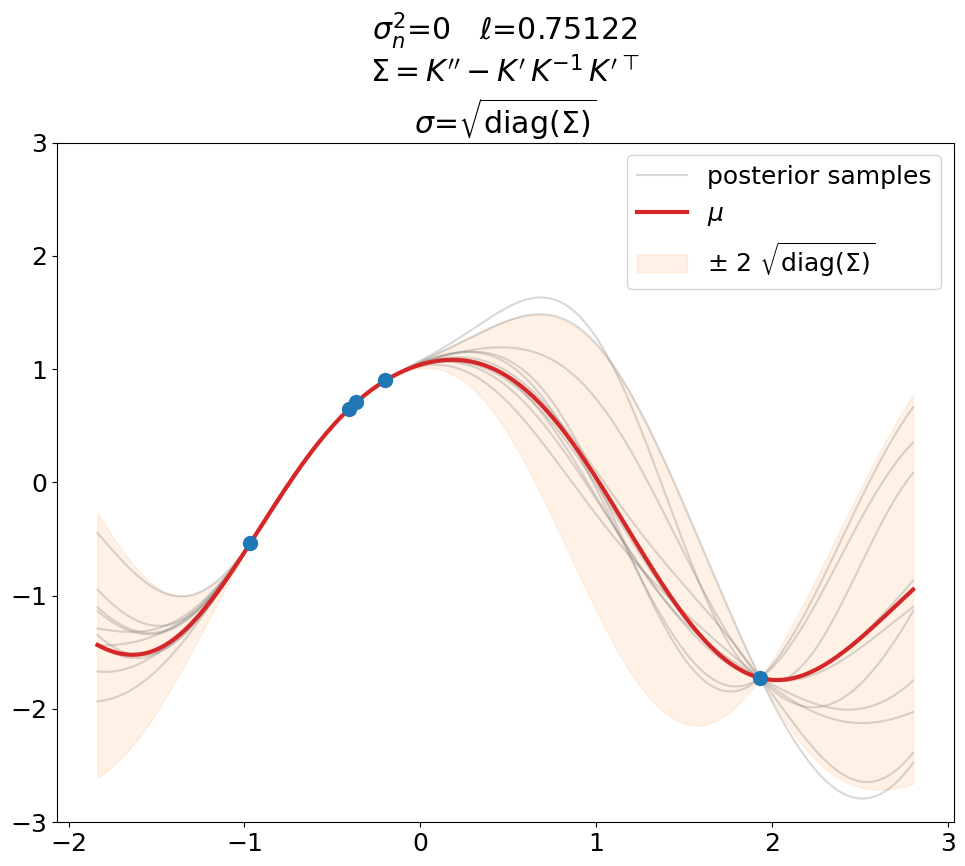
Posterior, noiseless, regression#
Regression (\(\sigma_n^2>0\)), predict_noiseless
.
You will see a plot like this also in text books. For instance
[Mur23] has this in fig.
18.7 (book version 2022-10-16). If you inspect the code that generates it
(which is open source, thanks!), you find that they use tinygp in the
predict_noiseless
setting.
Show code cell source
fig, ax = plt.subplots(figsize=figsize_single)
plot_gp_post(box=d_post_pn, ax=ax)
ax.legend(loc="upper right")
_ = ax.set_ylim(*y_lim)
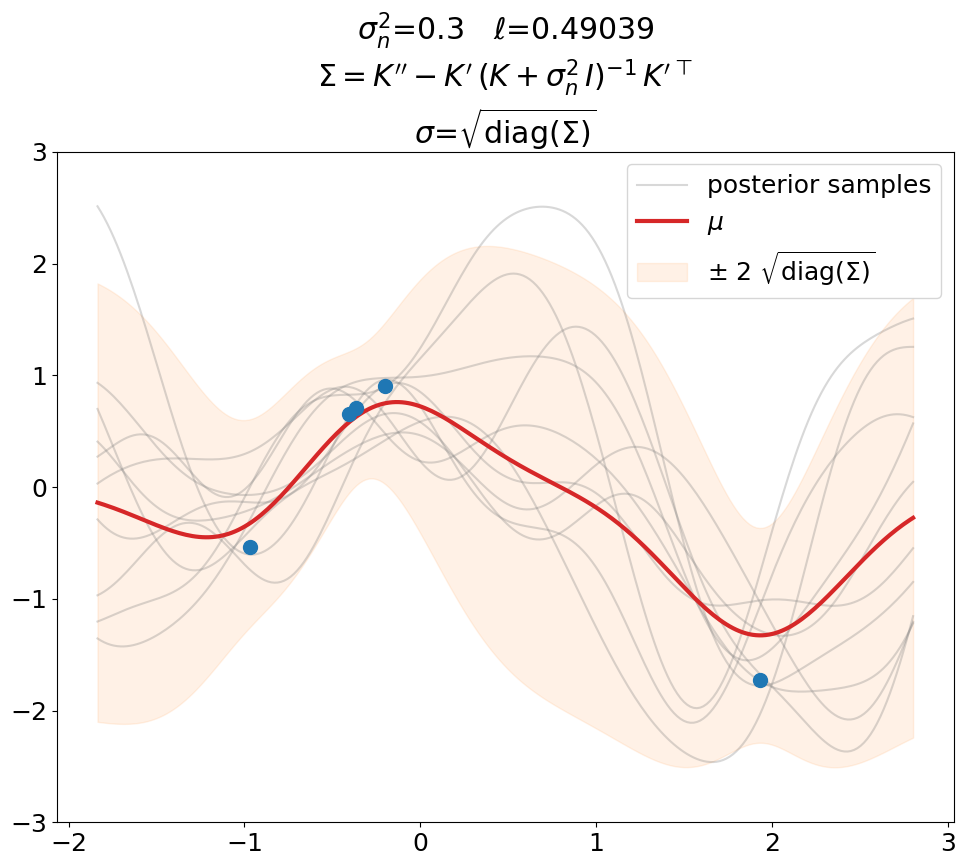
Posterior, noisy, regression#
Regression (\(\sigma_n^2>0\)), predict
.
This is the same as above in terms of optimized \(\ell\), \(\sigma_n^2\) value (fixed here, usually also optimized), fit weights \(\ve\alpha\) and thus predictions \(\ve\mu\).
The only difference is that we now use the covariance matrix \(\ma\Sigma + \sigma_n^2\,\ma I\) instead of \(\ma\Sigma\) to generate samples from the posterior. By that we get (1) noisy samples and (2) a larger \(\sigma\). That’s what is typically not shown in text books (at least not the ones we checked). Noisy samples (only for the prior, but still) are shown for instance in [DLvdW20] in fig. 4.
The difference in \(\sigma\) between predict
vs. predict_noiseless
is not
constant even though the constant \(\sigma_n^2\) is added to the diagonal
because of the \(\sqrt{\cdot}\) in
Show code cell source
fig, ax = plt.subplots(figsize=figsize_single)
plot_gp_post(box=d_post_p, ax=ax, std_color=y_std_p_color)
dd = d_post_pn
ax.fill_between(
xi,
dd.y_mean - 2 * dd.y_std,
dd.y_mean + 2 * dd.y_std,
alpha=0.1,
color=y_std_pn_color,
label=rf"$\pm$ 2 {dd.y_std_label}",
)
ax.legend(loc="upper right")
_ = ax.set_ylim(*y_lim)
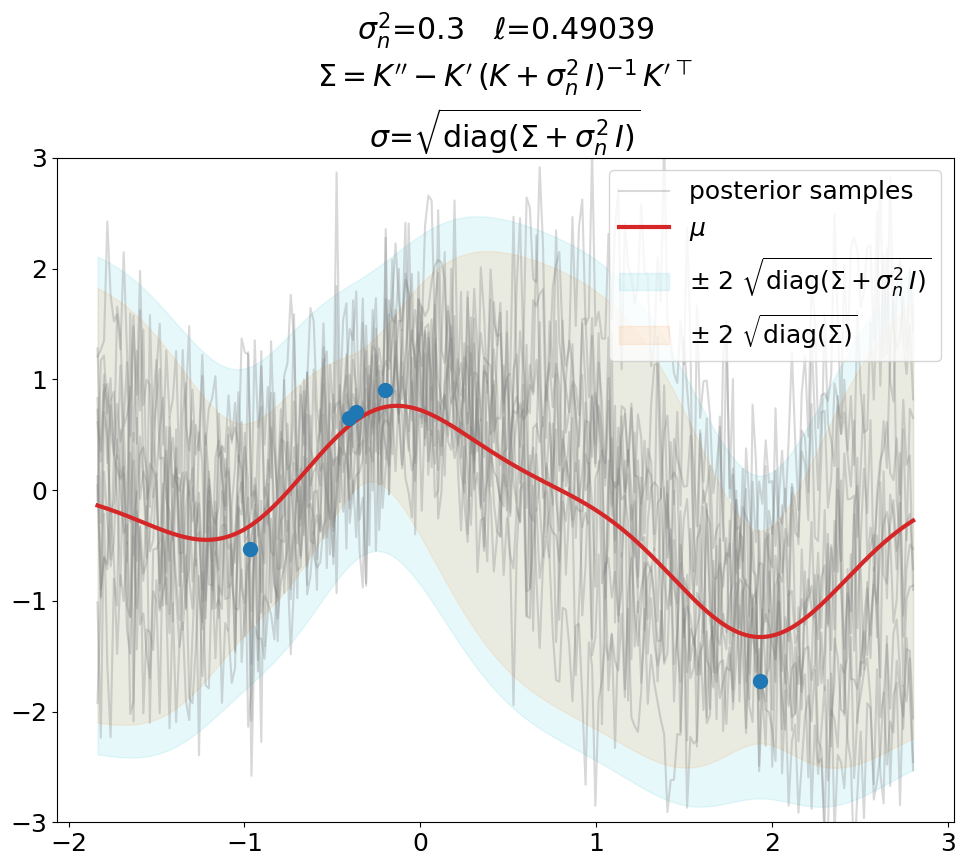
Show code cell source
fig, axs = plt.subplots(nrows=2, figsize=figsize_single)
for dd, color in [(d_post_p, y_std_p_color), (d_post_pn, y_std_pn_color)]:
axs[0].plot(xi, dd.y_std, label=dd.y_std_label, color=color)
aa = d_post_p
bb = d_post_pn
axs[1].plot(
xi, aa.y_std - bb.y_std, label=f"{aa.y_std_label} - {bb.y_std_label}"
)
for ax in axs:
ax.legend(loc="upper right")
# Compact plot in one fig.
#
##fig, axs = plt.subplots(
## nrows=3,
## ncols=3,
## gridspec_kw=dict(height_ratios=[1, 0.3, 0.3]),
## figsize=(30, 15),
## sharex=True,
##)
##
##
##plot_gp_post = partial(plot_gp, x=x, y=y, xi=xi)
##plot_gp_post(box=d_post_0, ax=axs[0, 0])
##plot_gp_post(box=d_post_pn, ax=axs[0, 1])
##plot_gp_post(box=d_post_p, ax=axs[0, 2], std_color=y_std_p_color)
##
##dd = d_post_pn
##axs[0, 2].fill_between(
## xi,
## dd.y_mean - 2 * dd.y_std,
## dd.y_mean + 2 * dd.y_std,
## alpha=0.1,
## color=y_std_pn_color,
## label=rf"$\pm$ 2 {dd.y_std_label}",
##)
##
##for dd, color in [(d_post_p, y_std_p_color), (d_post_pn, y_std_pn_color)]:
## axs[1, 2].plot(xi, dd.y_std, label=dd.y_std_label, color=color)
##
##aa = d_post_p
##bb = d_post_pn
##axs[2, 2].plot(
## xi, aa.y_std - bb.y_std, label=f"{aa.y_std_label} - {bb.y_std_label}"
##)
##
##for ax in axs[1:, :2].flat:
## ax.set_visible(False)
##
##for ax in [axs[0, 1], axs[0, 2], axs[1, 2], axs[2, 2]]:
## ax.legend(loc="upper right")
##
##for ax in axs[0, :]:
## ax.set_ylim(-3, 3)
##
##for ax in axs[1, :].flat:
## ax.set_ylim(-0.1, 1.3)
##
##for ax in axs[2, :].flat:
## ax.set_ylim(-0.1, 0.5)
### Make logo
##fig, ax = plt.subplots()
##plot_gp_post(box=d_post_pn, ax=ax, set_title=False)
##ax.set_axis_off()
##fig.savefig("logo.png", dpi=150)
if not is_interactive():
plt.show()
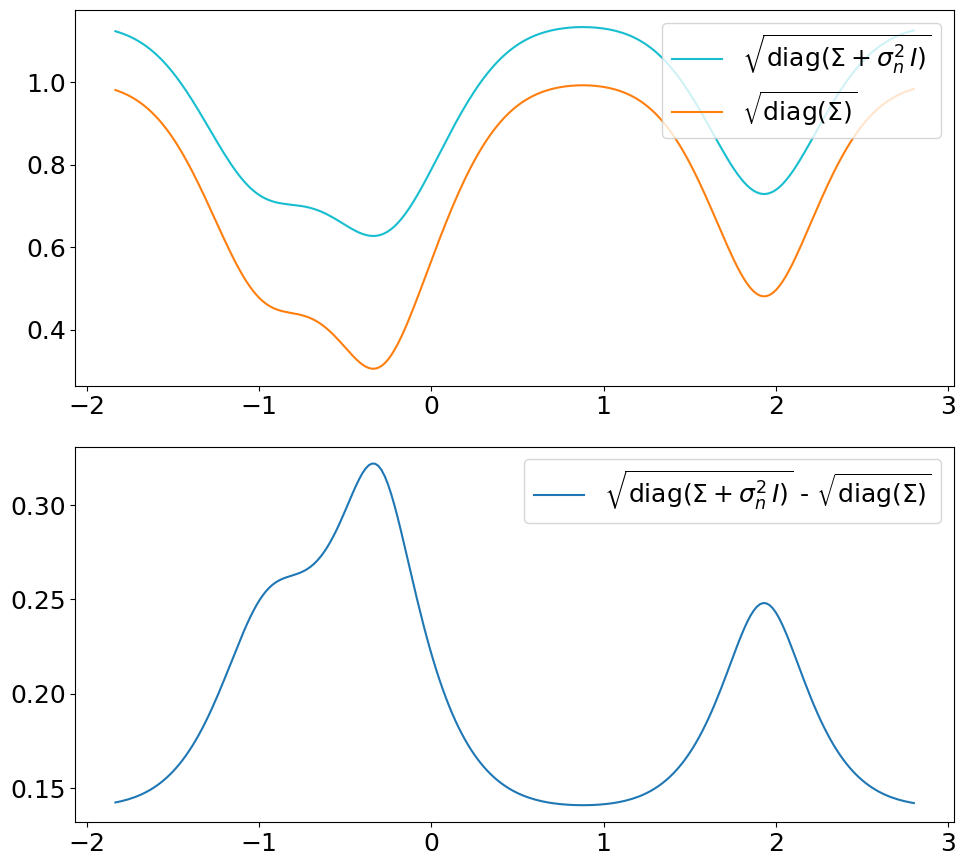